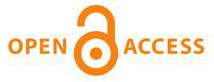
Address: Naberezhnaya Severnoy Dviny, 17, Arkhangelsk, 163002, Russian Federation, Northern (Arctic) Federal University named after M.V.Lomonosov, office 1425
Phone: +7 (8182) 21-61-18
E-mail: forest@narfu.ru
http://lesnoizhurnal.ru/en/
|
Applying the Random Forest Algorithm to Analyze the Dynamics of Taiga-Tundra Forest Ecosystems. С. 210-218
|
 |
Koptev S.V., Alabdullahalhasno H.
UDС
630*57
DOI:
10.37482/0536-1036-2025-2-210-218
Abstract
The article presents the results of studying the dynamics of taiga-tundra forest ecosystems in the Arkhangelsk Region based on the Earth remote sensing data and the application of image classification using the Random Forest algorithm. The change in the proportion of forested, non-forested and unforested areas in the study area is noted in the forest register. The results of the study show a significant increase in forest area between 2016 and 2023 (by 10.28 %) due to a reduction in non-forested areas and unstocked forest lands. This dynamics is due to the processes of successful natural restoration of non-forest covered areas, as well as their advancement northward taking-up lands due to climate change. The evaluation of the accuracy of automated classification of satellite images using the Random Forest algorithm by comparing them with reference data using criteria such as overall accuracy and the Kappa coefficient (the degree of correspondence between the model estimates and the actual data) has confirmed the reliability of the results obtained. The benchmarks have been taken from inventory databases, stationary sample plot data and the state forest inventory data. Before starting field work, cartographic databases have been studied and sample plots have been selected. Based on experimental data for the study area, a large number of polygons have been created, reflecting the diversity of forest stands and non-forest areas, to train the algorithm for classifying satellite images. Image processing, including corrections, mosaics, geoprojection and return, has been performed using SNAP (Sentinel Application Platform), an open source program. 100 points in various forest vegetation conditions in the study area have been analyzed. Studying the dynamics of forest ecosystems based on the Earth remote sensing data and the application of image classification using the Random Forest algorithm will improve the accuracy of assessing the resource and environmental potential of northern taiga and tundra forests of the Arkhangelsk Region.
Affiliation
2Northern Research Institute of Forestry, ul. Nikitova, 13, Arkhangelsk, 163062, Russian Federation; s.koptev@narfu.ru*
Keywordsdynamics of forest ecosystems, northern taiga forests, tundra forests, remote sensing, Random Forest algorithm
For citation
Koptev S.V., Alabdullahalhasno H. Applying the Random Forest Algorithm to Analyze the Dynamics of Taiga-Tundra Forest Ecosystems. Lesnoy Zhurnal = Russian Forestry Journal, 2025, no. 2, pp. 210–218. (In Russ.). https://doi.org/10.37482/0536-1036-2025-2-210-218
References
- Ilintsev A.S., Shamontyev I.G., Tretyakov S.V. Modern Dynamics of Forest Use in the Boreal Forests of Russia (For Example of the Arkhangelsk Region). Lesotekhnicheskij zhurnal = Forestry Engineering Journal, 2021, vol. 11, no. 3 (43), pp. 45–62. (In Russ.). https://doi.org/10.34220/issn.2222-7962/2020.3/4
- The State and Protection of the Environment in the Arkhangelsk Region for 2021: Report. The Centre for Nature Management and Environmental Protection, 2022, Arkhangelsk. 13 p. (In Russ.).
- Toskunina V.E. Problems of Timber Complex in the Arkhangelsk Region and Ways of Their Solution. Ekonomicheskie i sotsial’nye peremeny: fakty, tendentsii, prognoz = Economic and Social Changes: Facts, Trends, Forecast, 2008, iss. 3 (3), pp. 29–31. (In Russ.).
- Abdo W. Al-Din. Introduction to Remote Sensing and its Applications. University of Dammam, College of Architecture and Planning, 2014. 473 p.
- Arab Organization for Agricultural Development. Study of Modern Technologies Used in Developed Countries in the Field of Forest Conservation and Development. Khartoum, League of Arab States, 1998. 147 p.
- Barakat A., Khellouk R., El Jazouli A., Touhami F., Nadem S. Monitoring of Forest Cover Dynamics in Eastern Area of Béni-Mellal Province Using ASTER and Sentinel-2A Multispectral Data. Geology, Ecology and Landscapes, 2018, vol. 2, iss. 3, pp. 203–215. https://doi.org/10.1080/24749508.2018.1452478
- Du P., Samat A., Waske B., Liu S., Li Z. Random Forest and Rotation Forest for Fully Polarized SAR Image Classification Using Polarimetric and Spatial Features. ISPRS Journal of Photogrammetry and Remote Sensing, 2015, vol. 105, pp. 38–53. https://doi.org/10.1016/j.isprsjprs.2015.03.002
- FAO. The State of the World’s Forests 2022: Forest Pathways for Green Recovery and Building Inclusive, Resilient, and Sustainable Economies. Italy, Rome, FAO, 2022. 166 p. https://doi.org/10.4060/cb9360en
- Feizizadeh B., Darabi S., Blaschke T., Lakes T. QADI as a New Method and Alternative to Kappa for Accuracy Assessment of Remote Sensing-Based Image Classification. Sensors, 2022, vol. 22, no. 12, art. no. 4506. https://doi.org/10.3390/s22124506
- Foley J.A., DeFries R., Asner G.P., Barford C., Bonan G., Carpenter S.R., Chapin S., Coe M.T., Daily G.C., Gibbs H.K., Helkowski J.H., Holloway T., Howard E.A., Kucharik C.J., Monfreda C., Patz J.A., Prentice I.C., Ramankutty N., Snyder P.K. Global Consequences of Land Use. Science, 2005. vol. 309, iss. 5734, pp. 570–574. https://doi.org/10.1126/science.1111772
- Gebeyehu M.N. Remote Sensing and GIS Application in Agriculture and Natural Resource Management. International Journal of Environmental Sciences and Natutral Resourses, 2019, vol. 19, iss. 2, art. no. 556009. https://doi.org/10.19080/IJESNR.2019.19.556009
- Kalinaki K., Malik O.A., Lai D.T.C. FCD-AttResU-Net: An Improved Forest Change Detection in Sentinel-2 Satellite Images Using Attention Residual U-Net. International Journal of Applied Earth Observation and Geoinformation, 2023, vol. 122, art. no. 103453. https://doi.org/10.1016/j.jag.2023.103453
- Karra K., Kontgis C., Statman-Weil Z., Mazzariello J., Mathis M., Brumby S.P. Global Land Use / Land Cover with Sentinel 2 and Deep Learning. 2021 IEEE International Geoscience and Remote Sensing Symposium IGARSS. Belgium, Brussels, 2021, pp. 4704– 4707. https://doi.org/10.1109/IGARSS47720.2021.9553499
- Kayiranga A., Kurban A., Ndayisaba F., Nahayo L., Karamage F., Ablekim A., Li H., Ilniyaz O. Monitoring Forest Cover Change and Fragmentation Using Remote Sensing and Landscape Metrics in Nyungwe-Kibira Park. Journal of Geoscience and Environmental Protection, 2016, vol. 4, no. 11, pp. 13–33. https://doi.org/10.4236/gep.2016.411003
- Kuenzer C., Bluemel A., Gebhardt S., Quoc T.V., Dech S. Remote Sensing of Mangrove Ecosystems: A Review. Remote Sensing, 2011, vol. 3, no. 5, pp. 878–928. https://doi.org/10.3390/rs3050878
- Kumar D. Monitoring Forest Cover Changes Using Remote Sensing and GIS: A Global Prospective. Research Journal of Environmental Sciences, 2011, vol. 5, iss. 2, pp. 105–123. https://doi.org/10.3923/rjes.2011.105.123
- Lu D., Weng Q. A Survey of Image Classification Methods and Techniques for Improving Classification Performance. International Journal of Remote Sensing, 2007, vol. 28, iss. 5, pp. 823–870. https://doi.org/10.1080/01431160600746456
- Mangkhaseum Jr.S., Hanazawa A. Validation of Random Forest Algorithm to Monitor Land Cover Classification and Change Detection Using Remote Sensing Data in Google Earth Engine. Proceedings of SPIE - The International Society for Optical Engineering: Sensors and Smart Structures Technologies for Civil, Mechanical, and Aerospace Systems, 2022, vol. 12177, art. no. 121772L. https://doi.org/10.1117/12.2626085
- Mastrorosa S., Crespi M., Congedo L., Munafò M. Land Consumption Classification Using Sentinel 1 Data: A Systematic Review. Land, 2023, vol. 12, no. 4, art. no. 932. https://doi.org/10.3390/land12040932
- Mohajane M., Essahlaoui A., Oudija F., El Hafyani M., Teodoro A.C. Mapping Forest Species in the Central Middle Atlas of Morocco (Azrou Forest) through Remote Sensing Techniques. ISPRS International Journal of Geo-Information, 2017, vol. 6, no. 9, art. no. 275. https://doi.org/10.3390/ijgi6090275
- Mohammad H., Al-Bilbisi H., Abu Sammour H. Detection and Analysis of Vegetation Changes Using Spectral Botanical Indicators. Journal of Humanities and Social Sciences, 2018, vol. 45, no. 1, pp. 83–97
- Niemeyer J., Rottensteiner F., Soergel U. Contextual Classification of Lidar Data and Building Object Detection in Urban Areas. ISPRS Journal of Photogrammetry and Remote Sensing, 2014, vol. 87, pp. 152–165. https://doi.org/10.1016/j.isprsjprs.2013.11.001
- Panigrahy R.K., Kale M.P., Dutta U., Mishra A., Banerjee B., Singh S. Forest Cover Change Detection of Western Ghats of Maharashtra Using Satellite Remote Sensing Based Visual Interpretation Technique. Current Science, 2010, vol. 98, no. 5, pp. 657–664.
- Petrovska I., Dimov L. Accuracy Assessment of Unsupervised Land Cover Classification. Scientific Journal of Civil Engineering, 2020, vol. 9, iss. 2, pp. 83–88. https://doi.org/10.55302/sjce2092083p
- Praticò S., Solano F., Di Fazio S., Modica G. Machine Learning Classification of Mediterranean Forest Habitats in Google Earth Engine Based on Seasonal Sentinel-2 Time-Series and Input Image Composition Optimization. Remote Sensing, 2021, vol. 13, no. 4, art. no. 586. https://doi.org/10.3390/rs13040586
- Rodriguez-Galiano V.F., Ghimire B., Rogan J., Chica-Olmo M., Rigol-Sanchez J.P. An Assessment of the Effectiveness of a Random Forest Classifier for Land-Cover Classification. ISPRS Journal of Photogrammetry and Remote Sensing, 2012, vol. 67, pp. 93–104. https://doi.org/10.1016/j.isprsjprs.2011.11.002
- Turner II B.L., Lambin E.F., Reenberg A. The Emergence of Land Change Science for Global Environmental Change and Sustainability. PNAS, 2007, vol. 104, no. 52, pp. 20666–20671. https://doi.org/10.1073/pnas.0704119104
- Vanjare A., Omkar S.N., Senthilnath J. Satellite Image Processing for Land Use and Land Cover Mapping. International Journal of Image, Graphics and Signal Processing (IJIGSP), 2014, vol. 6, no. 10, pp. 18–28. https://doi.org/10.5815/ijigsp.2014.10.03
- Wang Y., Chen X. The Use of Random Forest to Identify Climate and Human Interference on Vegetation NDVI Changes in Southwest China. EGU General Assembly 2023. Austria, Vienna, 2023, art. no. EGU23-2315. https://doi.org/10.5194/egusphere-egu23-2315
- Zhou Y. Research on Forest Resource Change Detection Based on Decision Tree Algorithm. 2022 International Conference on Artificial Intelligence and Autonomous Robot Systems (AIARS). United Kingdom, Bristol, 2022, pp. 363–367. https://doi.org/10.1109/AIARS57204.2022.00088
Applying the Random Forest Algorithm to Analyze the Dynamics of Taiga-Tundra Forest Ecosystems. С. 210-218
|
Make a Submission
Lesnoy Zhurnal (Russian Forestry Journal) was awarded the "Seal of Recognition for Active Data Provider of the Year 2025"
|