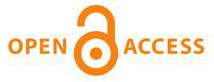
Address: Naberezhnaya Severnoy Dviny, 17, Arkhangelsk, 163002, Russian Federation, Northern (Arctic) Federal University named after M.V.Lomonosov, office 1425
Phone: +7 (8182) 21-61-18
E-mail: forest@narfu.ru
http://lesnoizhurnal.ru/en/
|
Assessment of the Transformation of Forests in the Southern Urals Based on Multi-Temporal Satellite Images. С. 51-62
|
 |
Sultanova R.R., Baiturina R.R., Diarova S.V.
UDС
630:528.88(470.57)
DOI:
10.37482/0536-1036-2025-2-51-62
Abstract
The transformation of forests in the Southern Urals over a 25-year period has been studied using geoinformation systems and remote sensing methods. The Normalized Difference Vegetation Index (NDVI) has been determined, which reflects the influence of such factors as chlorophyll content, leaf surface area, vegetation density and structure. The index has been calculated based on the interpretation of multi-temporal satellite images (1995, 2013 and 2020) from the Pleiades-1A, Landsat-5 and -8 satellites using the ArcGIS software package to create a vegetation map reflecting NDVI values and a retrospective analysis of the condition of forest vegetation. The values found have a close correlation with the vitality indicators obtained by the field method (the correlation coefficient is 0.69). The calculation of the NDVI index, the generation of maps of the range of fixed NDVI values and the combination of “artificial colours” channels by year has made it possible to identify forest areas with optimal density, age and sanitary condition (dark colour) and areas in need of reforestation. A comparison of the distribution of territory by NDVI classes for 1995–2020 indicates a significant change in the area of individual classes, which are grouped into 11 classes and are represented by values from –0.14 to +0.91. By 2020, the superior part of the study area has begun to belong to higher NDVI classes – 9 and 10, and has amounted to 98 %, which indicates the age uniformity of plantations and an increase in forest productivity since 1995. Plantations with higher NDVI indices are characterized by an increased accumulation of woody biomass compared to other forest stands. The territories of classes 1–9 (NDVI 0–0.8) demonstrate a significant reduction in area and similar dynamics, while the territories of grades 10–11 (NDVI 0.8–1.0) demonstrate noticeable positive dynamics. The results of the study confirm the correctness of choosing the NDVI index from the known vegetation indices for assessing the transformation of the underlying surface types of the study area over 25 years. Thus, this index is an objective indicator of the assessment of the condition of forest ecosystems. Spatial image interpretation methods can be used to determine the area of forested lands and significantly improve the efficiency of forest resource management.
Keywordsforest condition, forest condition change, remote sensing, geographic information system, vegetation index, underlying surface type, mapping, the Southern Urals
For citation
Sultanova R.R., Baiturina R.R., Diarova S.V. Assessment of the Transformation of Forests in the Southern Urals Based on Multi-Temporal Satellite Images. Lesnoy Zhurnal = Russian Forestry Journal, 2025, no. 2, pp. 51–62. (In Russ.). https://doi.org/10.37482/0536-1036-2025-2-51-62
References
- Kurbanov E.A., Lezhnin S.A., Vorobev O.N., Menshikov S.A., Dergunov D.M., Wang Y., Tarasova L.V., Smirnova L.N. Applications of Remote Sensing and GIS for Estimation of Land Use / Cover Change: A Review. Lesnye ekosistemy v usloviyakh izmeneniya klimata: biologicheskaya produktivnost’ i distantsionnyj monitoring = Forest Ecosystems in the Conditions of Climate Change: Biological Productivity, Monitoring and Technologies for Adaptation. Yoshkar-Ola, 2020, pp. 91–111. (In Russ.). https://doi.org/10.25686/7232.2020.6.58830
- Pin’kovskij M.D., Ivonin V.M., Samsonov S.D., Shiryaeva N.V., Egoshin A.V. Scientific Substantiation of the «Sochi National Park» GIS: Monograph. Sochi, Sole Proprietor Krivlyakin S.P. Printing House, 2011. 233 p. (In Russ.).
- Cherepanov A., Druzhinina E. Spectral Characteristics of Vegetation and Vegetation Indexes. Geomatika = Geomatics, 2009, no. 3, pp. 28–32. (In Russ.).
- Abbas S., Wong M.S., Wu J., Shahzad N., Irteza S.M. Approaches of Satellite Remote Sensing for the Assessment of Above-Ground Biomass across Tropical Forests: Pan-Tropical to National Scales. Remote Sensing, 2020, vol. 12, no. 20, art. no. 3351. https://doi.org/10.3390/rs12203351
- Ahrends A., Bulling M.T., Platts P.J., Swetnam R., Ryan C., Doggart N., Hollingsworth P.M., Marchant R., Balmford A., Harris D.J., Gross-Camp N., Sumbi P., Munishi P., Madoffe S., Mhoro B., Leonard C., Bracebridge C., Doody K., Wilkins V., Owen N., Marshall A.R., Schaafsma M., Pfilegner K., Jones T. Robinson J., Topp-Jørgensen E., Brink H., Burgess N.D. Detecting and Predicting Forest Degradation: A Comparison of Ground Surveys and Remote Sensing in Tanzanian Forests. Plants, People, Planet, 2021, vol. 3, iss. 3, pp. 268–281. https://doi.org/10.1002/ppp3.10189
- Arekhi M., Ylmaz O.Y., Yilmaz H., Akyüz Y.F. Can Tree Species Diversity Be Assessed with Landsat Data in a Temperate Forest? Environmental Monitoring and Assessment, 2017, vol. 189, art. no. 586. https://doi.org/10.1007/s10661-017-6295-6
- Ciesielski M., Sterenczak K. Volunteered Geographic Information data as a source of information on the use of forests in the Warsaw agglomeration // Sylwan, 2020, vol. 164, pp. 695–704.
- Féret J.-B., de Boissieu F. BiodivMapR: An R package for α- and β-Diversity Mapping Using Remotely Sensed Images. Methods in Ecology and Evolution, 2020, vol. 11, iss. 11, pр. 64–70. https://doi.org/10.1111/2041-210X.13310
- Fischer R., Knapp N., Bohn F., Huth A. Remote Sensing Measurements of Forest Structure Types for Ecosystem Service Mapping. Atlas of Ecosystem Services. Springer, Cham, 2019, pp. 63–67. https://doi.org/10.1007/978-3-319-96229-0_11
- Hepinstall-Cymerman J., Coe S., Alberti M. Using Urban Landscape Trajectories to Develop a Multi-Temporal Land Cover Database to Support Ecological Modeling. Remote Sensing, 2009, vol. 1, no. 4, pp. 1353–1379. https://doi.org/10.3390/rs1041353
- Hill M.J., Donald G.E. Estimating Spatio-Temporal Patterns of Agricultural Productivity in Fragmented Landscapes using AVHRR NDVI Time Series. Remote Sensing of Environment, 2003, vol. 84, iss. 3, pp. 367–384. https://doi.org/10.1016/S0034-4257 (02)00128-1
- Hycza T., Kamińska A., Stereńczak K. The Use of Remote Sensing Data to Estimate Land Area with Forest Vegetation Cover in the Context of Selected Forest Definitions. Forests, 2021, vol. 12, no. 11, art. no. 1489. https://doi.org/10.3390/f12111489
- Li Y., Li M., Li C., Liu Z. Forest Aboveground Biomass Estimation Using Landsat 8 and Sentinel-1A Data with Machine Learning Algorithms. Scientific Reports, 2020, vol. 10, art. no. 9952. https://doi.org/10.1038/s41598-020-67024-3
- Li Z., Chen H., White J.C., Wuder M.A., Hermosilla T. Discriminating Treed and Non-Treed Wetlands in Boreal Ecosystems Using Time Series Sentinel-1 Data. International Journal of Applied Earth Observation and Geoinformation, 2020, vol. 85, art. no. 102007. https://doi.org/10.1016/j.jag.2019.102007
- Loboda T., Krankina O., Savin I., Kurbanov E., Hall J. Land Management and the Impact of the 2010 Extreme Drought Event on the Agricultural and Ecological Systems of European Russia. Land–Cover and Land–Use Changes in Eastern Europe after the Collapse of the Soviet Union in 1991, Springer, Cham., 2017, pp. 173–192. https://doi.org/10.1007/978-3-319-42638-9_8
- Mao F., Du H., Li X., Ge H., Cui L., Zhou G. Spatiotemporal Dynamics of Bamboo Forest Net Primary Productivity with Climate Variations in Southeast China. Ecological Indicators, 2020, vol. 116, art. no. 106505. https://doi.org/10.1016/j.ecolind.2020.106505
- Nguyen H.-H., McAlpine C., Pullar D., Johansen K., Duke N.C. The Relationship of Spatial–Temporal Changes in Fringe Mangrove Extent and Adjacent Land-Use: Case Study of Kien Giang Coast, Vietnam. Ocean & Coastal Management, 2013, vol. 76, pp. 12–22. https://doi.org/10.1016/j.ocecoaman.2013.01.003
- Pechanec V., Štěrbová L., Purkyt J., Prokopová M., Včeláková R., Cudlin O., Vyvlečka P., Cienciala E., Cudlin P. Selected Aspects of Carbon Stock Assessment in Aboveground Biomass, 2022, vol. 11, no. 1, art. no. 66. https://doi.org/10.3390/land11010066
- Sellers P.J., Tucker C.J., Collatz G.J., Los S.O., Justice C.O., Dazlich D.A., Randall D.A. A Global 1° by 1° NDVI Data Set for Climate Studies. Part 2: The Generation of Global Fields of Terrestrial Biophysical Parameters from the NDVI. International Journal of Remote Sensing, 1994, vol. 15, iss. 17, pp. 3519–3545. https://doi.org/10.1080/01431169408954343
- Townshend J.R.G., Justice C.O., Skole D., Malingreau J.-P., Cihlar J., Teillet P., Sadowski F., Ruttenberg S. The 1 km Resolution Global Data Set: Needs of the International Geosphere Biosphere Programme. International Journal of Remote Sensing, 1994, vol. 15, iss. 17, pp. 3417–3441. https://doi.org/10.1080/01431169408954338
- Valderrama-Landeros L., Flores-Verdugo F., Rodríguez-Sobreyra R., Kovacs J.M., Flores-de-Santiago F. Extrapolating Canopy Phenology Information Using Sentinel-2 Data and the Google Earth Engine Platform to Identify the Optimal Dates for Remotely Sensed Image Acquisition of Semiarid Mangroves. Journal of Environmental Management, 2021, vol. 279, art. no. 111617. https://doi.org/10.1016/j.jenvman.2020.111617
- Wang Q., Watanabe M., Hayashi S., Murakami S. Using NOAA AVHRR Data to Assess Flood Damage in China. Environmental Monitoring and Assessment, 2003, vol. 82, pp. 119–148. https://doi.org/10.1023/A:1021898531229
- Wani A.A., Bhat A.F., Gatoo A.A., Zahoor S., Mehraj B., Najam N., Wani Q.S., Islam M.A., Murtaza S., Dervash M.A., Joshi P.K. Assessing Relationship of Forest Biophysical Factors with NDVI for Carbon Management in Key Coniferous Strata of Temperate Himalayas. Mitigation and Adaptation Strategies for Global Change, 2021, vol. 26, art. no. 1. https://doi.org/10.1007/s11027-021-09937-6
- Wiggins H.L., Nelson C.R., Larson A.J., Safford H.D. Using LiDAR to Develop High-Resolution Reference Models of Forest Structure and Spatial Pattern. Forest Ecology and Management, 2019, vol. 434, pp. 318–330. https://doi.org/10.1016/j.foreco.2018.12.012
- Wolter P.T., Townsend P.A., Sturtevant B.R. Estimation of Forest Structural Parameters Using 5 and 10 Meter SPOT-5 Satellite Data. Remote Sensing of Environment, 2009, vol. 113, iss. 9, pp. 2019–2036. https://doi.org/10.1016/j.rse.2009.05.009
- Yasmin N., Khokhar M.F., Tanveer S., Saqib Z., Khan W.R. Dynamical Assessment of Vegetation Trends over Margalla Hills National Park by Using MODIS Vegetation Indices. Pakistan Journal of Agricultural Sciences, 2016, vol. 53 (4), pp. 777–786. https://doi.org/10.21162/PAKJAS/16.3759
Assessment of the Transformation of Forests in the Southern Urals Based on Multi-Temporal Satellite Images. С. 51-62
|
Make a Submission
Lesnoy Zhurnal (Russian Forestry Journal) was awarded the "Seal of Recognition for Active Data Provider of the Year 2025"
|