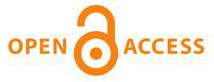
Address: 17 Naberezhnaya Severnoy Dviny, Arkhangelsk 163002 Russian Federation. Northern (Arctic) Federal University named after M.V.Lomonosov. Office 1425
Phone / Fax: (818-2) 21-61-18
E-mail: forest@narfu.ru
http://lesnoizhurnal.ru/en/

|
Forest Exploitation Zoning of Forest Subarctic Territories. C. 114-135
|
 |

These works are licensed under a Creative Commons Attribution 4.0 International License.
P.V. Budnik, V.N. Baklagin, O.N. Galaktionov
UDС
630*0
DOI:
10.37482/0536-1036-2023-6-114-135
Abstract
The purpose of this study is to classify the territories of the forest fund of the Republic of Karelia and Murmansk region at the level of central forestries on the basis of multifactorial assessment of timber resources, natural and production conditions, and road infrastructure. Forest exploitation zoning was carried out taking into account 20 variables. For this purpose, factor and hierarchical cluster analysis, k-means method and discriminant analysis were successively applied. Based on statistical methods, all central forestries were distributed into 9 clusters: 4 large clusters (No. 2, 3, 8, 9), including several forestries, and 5 (No. 1, 4–7), each formed by one forestry. Forestries of cluster No. 2 had large annual allowable cut (AAC), significant average forest reserve per hectare and hardwood reserve. Forestries of cluster No. 3 were characterized by small AAC and forest reserves per hectare. Cluster No. 8 forestries were characterized by average AAC and forest stock per hectare, high proportion of forests in stagnant moisture conditions. Forestries of cluster No. 9 were intermediate in their characteristics between the forestries of clusters No. 2 and No. 8. The forestry of cluster No. 1 is close to the forestry of cluster No. 2 in its characteristics, but its forests are more productive. Forestries of small clusters No. 4, 6, 7 have the highest forest stock per hectare. Differences in some variables did not allow them to be combined into one cluster. The forestry of cluster No. 5 is similar in terms of natural and production conditions to the forestry of cluster No. 3 but had a small estimated harvesting area for the coniferous component. The results of the study can be considered when solving the issues of organization and development of forestry and forest industry complex in the Republic of Karelia and Murmansk region.
Affiliation
1Petrozavodsk State University, prosp. Lenina, 33, Petrozavodsk, 185910, Russian Federation; budnikpavel@yandex.ru*, ong66@mail.ru
2Northern Water Problems Institute of the Karelian Research Centre of the Russian Academy of Sciences, prosp. Alexandra Nevskogo, 50, Petrozavodsk, 185030, Russian Federation; slava.baklagin@mail.ru
Keywordszoning, factor analysis, cluster analysis, k-means method, discriminant analysis, logging, forest management
For citation
Budnik P.V., Baklagin V.N., Galaktionov O.N. Forest Exploitation Zoning of Forest Subarctic Territories. Lesnoy Zhurnal = Russian Forestry Journal, 2023, no. 6, pp. 114–135. (In Russ.). https://doi.org/10.37482/0536-1036-2023-6-114-135
References
- Pasko O.A., Zakharchenko A.V., Kovyazin V.F. Analysis of Land Management of the Forest Fund. Bulletin of the Tomsk Polytechnical University, 2021, vol. 332, no. 2, pp. 127–138. (In Russ.). https://doi.org/10.18799/24131830/2021/2/3049
- Sukachev V.N. Selected Works, vol. 1. Fundamentals of Forest Typology and Biogeocenology. Leningrad, Nauka Publ., 1972. 418 p. (In Russ.).
- Khlyustov V.K., Ganikhin A.M., Korotkaya S.V. Resource and Ecological Zoning of Forests of the Nizhny Novgorod Region. Prirodoobustroystvo, 2021, no. 3, pp. 128–136. (In Russ.). https://doi.org/10.26897/1997-6011-2021-3-128-136
- Coates A., Ng A.Y. Learning Feature Representations with K-Means. Neural Networks: Tricks of the Trade, Springer, Berlin, Heidelberg, 2012, vol. 7700, pp. 561–580. https://doi.org/10.1007/978-3-642-35289-8_30
- Cochran W.G., Snedecor G.W. Statistical methods. Iowa State University Press, 1980. 507 p.
- DeArmond D., Ferraz João B.S., Higuchi N. Natural Recovery of Skid Trails: a Review. Canadian Journal of Forest. Research, 2021, vol. 51, no. 7, pp. 948–961. https://doi.org/10.1139/cjfr-2020-0419
- Eliasson L., Bengtsson J., Cedergren J., Lageson H. Comparison of Single-Grip Harvester Productivity in Clearand Shelterwood Cutting. Journal of Forest Engineering, 1999, no. 10, pp. 43–48.
- Gendler S., Prokhorova E. Risk-Based Methodology for Determining Priority Directions for Improving Occupational Safety in the Mining Industry of the Arctic Zone. Resources, 2021, vol. 10, no. 3, art. 20. https://doi.org/10.3390/resources10030020
- George A.K., Kizha A.R., Kenefic L. Economic Feasibility of Timber Harvesting in Lowlands. Exceeding the Vision: Forest Mechanisation of the Future. Proceedings of the 52nd International Symposium on Forestry Mechanization. Sopron, Hungary, University of Sopron Press, 2019, pp. 379–393.
- Gerasimov Y., Sokolov A.P., Fjeld D. Improving Cut-to-Length Operations Management in Russian Logging Companies Using a New Decision Support System. Baltic Forestry, 2013, vol. 19, no. 1, pp. 89–105.
- Goodsite M., Swanström N. Towards a Sustainable Arctic. World Scientific Publishing Europe Ltd., 2023. 280 p. https://doi.org/10.1142/q0390
- Härdle W.K., Simar L. Applied Multivariate Statistical Analysis. Berlin, Germany, Springer Cham Publ., 2019. 559 p. https://doi.org/10.1007/978-3-030-26006-4
- Hartwell C.A. In Our (Frozen) Backyard: the Eurasian Union and Regional Environmental Governance in the Arctic. Climatic Change, 2023, vol. 176, art. 45. https://doi.org/10.1007/s10584-023-03491-7
- Hiesl P., Benjamin J.G. Applicability of International Harvesting Equipment Productivity Studies in Maine, USA: A Literature Review. Forests, 2013, vol. 4, iss. 4, pp. 898–921. https://doi.org/10.3390/f4040898
- Ilintsev A., Nakvasina E., Högbom L., Bogdanov A. Influence of Ruts on the Physical Properties of Gleyic Retisols After Logging Machinery Passage. Scandinavian Journal of Forest Research, 2022, vol. 37, iss. 4, pp. 254–263. https://doi.org/10.1080/02827581.2022.2085785
- Jaeger A., Banks D. Cluster analysis: A modern statistical review. WIREs Computational Statistics, 2023, vol. 15, iss. 3, art. 1597. https://doi.org/10.1002/wics.1597
- Kaiser H.F. The Application of Electronic Computers to Factor Analysis. Educational and Psychological Measurement, 1960, vol. 20, iss. 1, pp. 141–151. https://doi.org/10.1177/001316446002000116
- Kaiser H.F. The Varimax Criterion for Analytic Rotation in Factor Analysis. Psychometrika, 1958, vol. 23, pp. 187–200. https://doi.org/10.1007/BF02289233
- Kaiser H.F., Rice J. Little Jiffy, Mark IV. Educational and Psychological Measurement, 1974, vol. 34, iss. 1, pp. 111–117. https://doi.org/10.1177/001316447403400115
- Kärhä K., Rönkkö E., Gumse S.I. Productivity and Cutting Costs of Thinning Harvesters. International Journal of Forest Engineering, 2004, vol. 15, iss. 2, pp. 43–56. https://doi.org/10.1080/14942119.2004.10702496
- Kellogg L.D., Bettinger P. Thinning Productivity and Cost for Mechanized CuttoLength System in the Northwest Pacific Coast Region of the USA. Journal of Forest Engineering, 1994, vol. 5, iss. 2, pp. 43–54.
- Klaes B., Struck J., Schneider R., Schueler G. Middle-term Effects After Timber Harvesting with Heavy Machinery on a Fine-Textured Forest Soil. European Journal of Forest Research, 2016, vol. 135, iss. 6, pp. 1083–1095.
- Kormanek M., Baj D. Analysis of Operation Performance in the Process of Machine Wood Harvesting with Fao Far 6840 Mini-Harvester. Agricultural engineering, 2018, vol. 22, iss. 1, pp. 73–82.
- Labelle E.R., Soucy M. Effect of Tree Form on the Productivity of a Cut-to-Length Harvester in a Hardwood Dominated Stand. Croatian Journal of Forest Engineering, 2016, vol. 37, iss. 1, pp. 175–183.
- Liubachyna A., Bubbico A., Secco L., Pettenella D. Management Goals and Performance: Clustering State Forest Management Organizations in Europe with Multivariate Statistics. Forests, 2017, vol. 8, iss. 12, art. 504. https://doi.org/10.3390/f8120504
- Louis L.T., Kizha A.R., Daigneault A., Han H.S., Weiskittel A. Factors Affecting Operational Cost and Productivity of Ground-Based Timber Harvesting Machines: a Meta-analysis. Current Forestry Reports, 2022, vol. 8, pp. 38–54. https://doi.org/10.1007/s40725-021-00156-5
- Meira Castro A.C., Nunes A., Sousa A., Lourenço L. Mapping the Causes of Forest Fires in Portugal by Clustering Analysis. Geosciences, 2020, vol. 10, iss. 2, art. 53. https://doi.org/10.3390/geosciences10020053
- Melchiori L., Nasini G., Montagna J.M., Corsano G. A Mathematical Modeling for Simultaneous Routing and Scheduling of Logging Trucks in the Forest Supply Chain. Forest Policy and Economics, 2022, vol. 136, art. 102693. https://doi.org/10.1016/j.forpol.2022.102693
- Mokhirev A., Medvedev S. Assessment of Road Density in Logging Areas Using Geographical Information Systems. IOP Conference Series: Earth and Environmental Science, All-Russian scientific-technical conference “Digital technologies in forest sector”, 26–27 March 2020, Saint Petersburg, Russian Federation, vol. 507, art. 012022. https://doi.org/10.1088/1755-1315/507/1/012022
- Muttilainen H., Hallikainen V., Miina J., Vornanen J., Vanhanen H. Forest Owners’ Perspectives Concerning Non-Timber Forest Products, Everyman’s Rights, and Organic Certification of Forests in Eastern Finland. Small-scale Forestry, 2023, vol. 22, pp. 69–101. https://doi.org/10.1007/s11842-022-09528-6
- Olivera A., Visser R., Acuna M., Morgenroth J. Automatic GNSS-enabled Harvester Data Collection as a Tool to Evaluate Factors Affecting Harvester Productivity in a Eucalyptus Spp. Harvesting Operation in Uruguay. International Journal of Forest Engineering, 2016, vol. 27, iss. 1, pp. 15–28. https://doi.org/10.1080/14942119.2015.1099775
- Parente J., Pereira M.G., Tonini M. Space-Time Clustering Analysis of Wildfires: the Influence of Dataset Characteristics, Fire Prevention Policy Decisions, Weather and Climate. Science of the Total Environment, 2016, vol. 559, pp. 151–165. https://doi.org/10.1016/j.scitotenv.2016.03.129
- Proto A.R., Macrì G., Visser R., Harrill Н., Russo D., Zimbalatti G. Factors Affecting Forwarder Productivity. European Journal of Forest Research, 2018, vol. 137, pp. 143–151. https://doi.org/10.1007/s10342-017-1088-6
- Salimova G., Ableeva A., Lubova T., Sharafutdinov A., Araslanbaev I. Multidimensional Modeling of the Economy of Forest Management and Reforestation. Ecological Modelling, 2022, vol. 472, art. 110098. https://doi.org/10.1016/j.ecolmodel.2022.110098
- Sccoti M.S.V., Mascarenhas A.R.P., Rebelo A.C., Fernandes I.M., Vendruscolo J., Rocha J.D.S., Moreto R.F. Current Practices of Conducting Forest Management Plans in the Amazon May Risk the Survival of Timber Species. Environmental Science and Pollution Research, 2023, vol. 30, pp. 82589–82600. https://doi.org/10.1007/s11356-023-28311-4
- Schreiber J.B. Issues and Recommendations for Exploratory Factor Analysis and Principal Component Analysis. Research in Social and Administrative Pharmacy, 2021, vol. 17, iss. 5, pp. 1004–1011. https://doi.org/10.1016/j.sapharm.2020.07.027
- Senko S. Nordic Forest Solutions as an Opportunity to Reform the Forestry Sector in Russia: A Case Study in the Republic of Karelia. Dissertationes Forestales, 2021, vol. 320. 61 p. https://doi.org/10.14214/df.320
- Shabaev A., Sokolov A., Urban A., Pyatin D. Optimal Planning of Wood Harvesting and Timber Supply in Russian Conditions. Forests, 2020, vol. 11, iss. 6, art. 662. https://doi.org/10.3390/f11060662
- Shegelman I., Budnik P., Baklagin V., Galaktionov O., Khyunninen I., Popov A. Analysis of Natural-Production Conditions for Timber Harvesting in European North of Russia. Central European Forestry Journal, 2019, vol. 65, iss. 2, pp. 81–91.
- Spinelli К., Magagnotti N. The Effect of Harvest Tree Distribution on Harvesting Productivity in Selection Cuts. Scandinavian Journal of Forest Research, 2013, vol. 28, iss. 7, pp. 701–709. https://doi.org/10.1080/02827581.2013.821517
- Tutmez B., Ozdogan M.G., Boran A. Mapping Forest Fires by Nonparametric Clustering Analysis. Journal of Forestry Research, 2018, vol. 29, pp. 177–185. https://doi.org/10.1007/s11676-017-0417-4
- Varol T., Emir T., Akgul M., Ozel H.B., Acar H.H., Cetin M. Impacts of SmallScale Mechanized Logging Equipment on Soil Compaction in Forests. Journal of Soil Science and Plant Nutrition, 2020, vol. 20, pp. 953–963. https://doi.org/10.1007/s42729-020-00182-5
- Zykov S.V., Dayneko D.V. The Analysis of the Russian Forest Industry. Forest Industry of Russia. Singapore, Springer Publ., 2022, pp. 17–103. https://doi.org/10.1007/978-981-16-9861-3_2
Forest Exploitation Zoning of Forest Subarctic Territories. C. 114-135
|
Make a Submission
Lesnoy Zhurnal (Russian Forestry Journal) was awarded the "Seal of Recognition for Active Data Provider of the Year 2025"
|